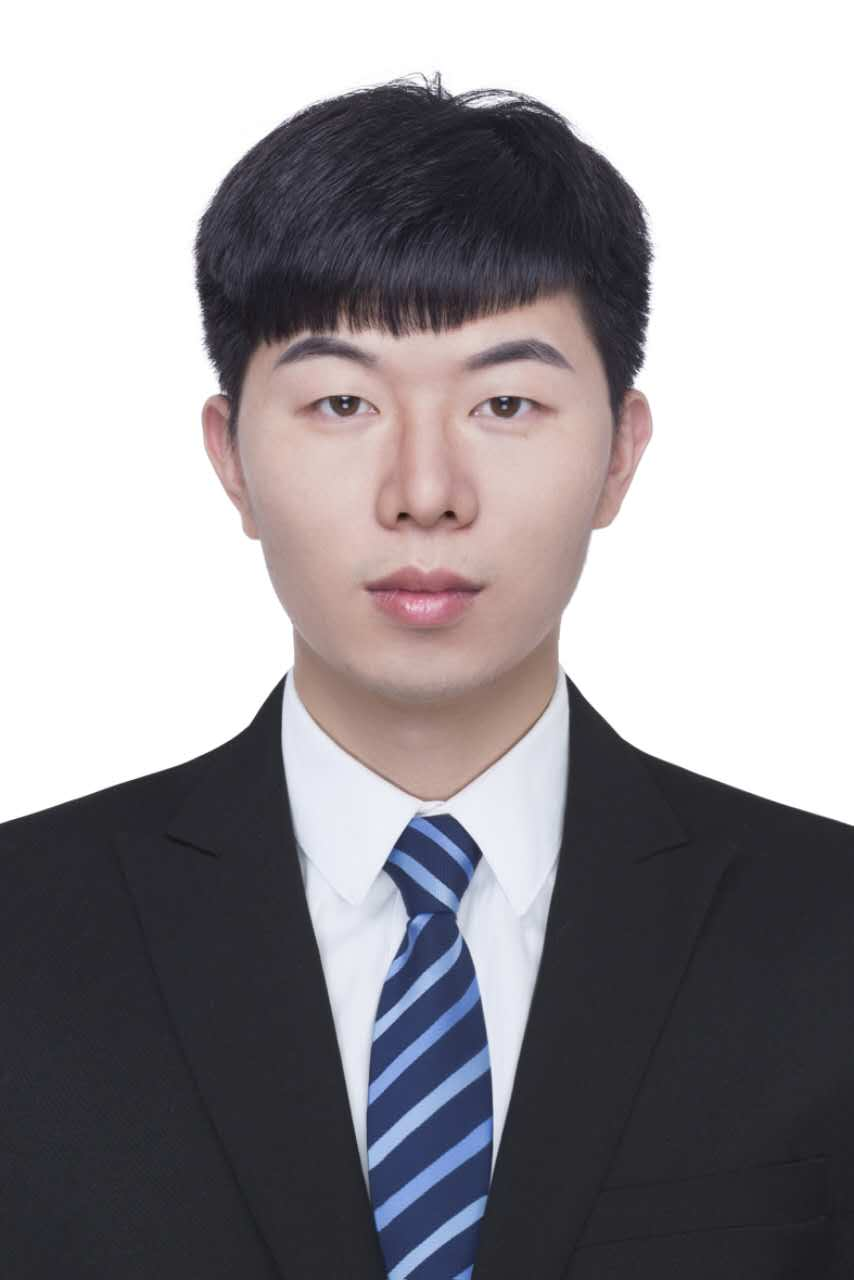
Yan, Qin, Professor of Chongqing University, China.
Yan Qin received the B.S. degree in electronic information engineering from Information Engineering University, Zhengzhou, China, in 2011, the M.S. degree in control theory and control engineering from Northeastern University, Shenyang, China, in 2013, and the Ph.D. degree in control science and engineering from Zhejiang University, Hangzhou, China, in 2018. From 2019 to 2022, he was a Post-Doctoral Research Fellow with the Singapore University of Technology and Design, Singapore, and the Nanyang Technological University, Singapore. He is currently the Professor of the School of Automation, Chongqing University, Chongqing, China. His research interests include data-driven process monitoring, soft sensor modeling, and remaining useful life estimation for industrial processes and essential assets.
Contact Information
Email: yan.qin@cqu.edu.cn
Address: Shazheng Street174#, Shapingba District, Chongqing, 400044, China
Education
Ph.D., (E.E.), Zhejiang University, P. R. China, 2018.
M.S., (E.E.), Northeastern University, P. R. China, 2013.
Academic Experience
Professor, 11/2023- present, Chongqing University, Chongqing, P. R. China
Associate Professor, 03/2023-10/2023, Chongqing University, Chongqing, P. R. China
Research Interests
Data-driven process monitoring
Soft sensor modeling
Remaining useful life estimation
Publications (selected)
[1] Anushiya Arunan, Yan Qin*, Xiaoli Li, and Chau Yuen*. A federated learning-based industrial health prognostics for heterogeneous edge devices using matched feature extraction [J]. IEEE Transactions on Automation Science and Engineering. Accepted, 2023.
[2] K.Q. Zhou, Y. Qin*, and C. Yuen*. Lithium-ion battery online knee onset detection by matrix profile [J]. IEEE Transactions on Transportation Electrification. Early Access, 2023.
[3] Y. Qin, Yuen Chau*, Yongliang Guan. Capsule neural network enabled vehicle trajectory prediction in the V2X network [J]. IEEE Transaction on Vehicular Technology. Early Access, 2023.
[4] Y. Qin, A. Auran, C. Yuen*. Digital twin for real-time Li-ion battery state of health estimation with partially discharged cycling data [J]. IEEE Transaction on Information Informatics. In press, 2023.
[5] Chintaka, Y. Qin*, C. Yuen, and et al. A hybrid deep learning model based remaining useful life estimation for reed relay with degradation pattern clustering [J]. IEEE Transaction on Information Informatics, Early Access, 2022.
[6] Y. Qin, C. Yuen*, X. Yin, H. Biao. A transferable multi-stage model with cycling discrepancy learning for Lithium-ion battery state of health estimation [J]. IEEE Transaction on Information Informatics, In press, 2022, DOI: 10.1109/TII.2022.3205942.
[7] K.Q. Zhou, Y. Qin*, and C. Yuen. Transfer learning-based state of health estimation for Lithium-ion battery with cycle synchronization [J]. IEEE/ASME Transactions on Mechatronics, In press, 2022, DOI: 10.1109/TMECH.2022.3201010.
[8] Y. Qin, C. Yuen*, Y.M. Shao, B. Qin, and X. Li. Slow-varying dynamics-assisted temporal capsule network for machinery remaining useful life estimation [J]. IEEE Transaction on Cybernetics, vol. 53, no. 1, pp. 592-606, Jan. 2023.
[9] Y. Qin*, W. Li, C. Yuen, W. Tushar, and T.K. Saha. IIoT-enabled health monitoring for integrated heat pump system using mixture slow feature analysis [J]. IEEE Transaction on Information Informatics, vol. 18, no. 7, pp. 4725-4736, 2022.
[10] Y. Qin*, S. Adams, and C. Yuen. A transfer learning-based state of charge estimation for Lithium-ion battery at varying ambient temperatures [J]. IEEE Transaction on Information Informatics, vol. 17, no. 11, pp. 7304-7315, 2021.
[11] Y. Qin, C.H. Zhao. A comprehensive process decomposition based on quality-relevant slow feature regression for soft sensor modelling [J]. Journal of Process Control. 2019. 77: 141-154.
[12] Y. Qin, C.H. Zhao, B. Huang. A new soft-sensor algorithm with concurrent consideration of slowness and quality interpretation for dynamic process [J]. Chemical Engineering Science. 2019. 199: 28-39.
[13] Y. Qin, C.H. Zhao, F.R. Gao. An intelligent non-optimality self-recovery method based on reinforcement learning with small data in big data era [J]. Chemometrics and Intelligent Laboratory Systems. 2018. 176: 89-100.
[14] Y. Qin, C.H. Zhao, F.R. Gao. An iterative two-step sequential phase partition (ITSPP) method for batch process modelling and online monitoring [J]. AIChE Journal. 2016. 62(7): 2358-2373.